Cloud Parquet ETL with Dask DataFrame#
This notebook looks at every Uber and Lyft ride in New York City over the last several years. Because the data is large, we operate on a cluster in the cloud.
Install Dask and Coiled#
This example requires the packages dask s3fs matplotlib coiled
. We do this in a new virtual environment below, but you could also install them in whatever environment you’re already using.
conda create -n coiled-nyc -c conda-forge python=3.11 coiled dask s3fs matplotlib
conda activate coiled-nyc
You also could use pip
for everything, or any other package manager you prefer. conda
isn’t required.
When you later create a Coiled cluster, your local coiled-nyc
environment will be automatically replicated on your cluster.
Create Dask cluster in the cloud#
This dataset is ~100 GB in size, and so is too large for a typical laptop. We use Coiled to create a Dask cluster that’s big enough to handle our data comfortably.
import coiled
cluster = coiled.Cluster(
n_workers=30,
region="us-east-2", # Start workers in same region as data to minimize costs
)
client = cluster.get_client()
This is the only Coiled-specific code. The remainder of this example is normal Dask code that would look the same for any Dask deployment.
Load and prepare data#
Let’s look at our data using Dask DataFrame’s read_parquet
function to load our dataset from S3.
import dask.dataframe as dd
df = dd.read_parquet("s3://coiled-data/uber/")
df.head()
hvfhs_license_num | dispatching_base_num | originating_base_num | request_datetime | on_scene_datetime | pickup_datetime | dropoff_datetime | PULocationID | DOLocationID | trip_miles | ... | sales_tax | congestion_surcharge | airport_fee | tips | driver_pay | shared_request_flag | shared_match_flag | access_a_ride_flag | wav_request_flag | wav_match_flag | |
---|---|---|---|---|---|---|---|---|---|---|---|---|---|---|---|---|---|---|---|---|---|
0 | HV0003 | B02867 | B02867 | 2019-02-01 00:01:26 | 2019-02-01 00:02:55 | 2019-02-01 00:05:18 | 2019-02-01 00:14:57 | 245 | 251 | 2.45 | ... | 0.83 | 0.0 | NaN | 0.0 | 7.480000 | Y | N | N | N | NaN |
1 | HV0003 | B02879 | B02879 | 2019-02-01 00:26:08 | 2019-02-01 00:41:29 | 2019-02-01 00:41:29 | 2019-02-01 00:49:39 | 216 | 197 | 1.71 | ... | 0.70 | 0.0 | NaN | 2.0 | 7.930000 | N | N | N | N | NaN |
2 | HV0005 | B02510 | <NA> | 2019-02-01 00:48:58 | NaT | 2019-02-01 00:51:34 | 2019-02-01 01:28:29 | 261 | 234 | 5.01 | ... | 3.99 | 0.0 | NaN | 0.0 | 35.970001 | N | Y | N | N | NaN |
3 | HV0005 | B02510 | <NA> | 2019-02-01 00:02:15 | NaT | 2019-02-01 00:03:51 | 2019-02-01 00:07:16 | 87 | 87 | 0.34 | ... | 0.64 | 0.0 | NaN | 3.0 | 5.390000 | N | Y | N | N | NaN |
4 | HV0005 | B02510 | <NA> | 2019-02-01 00:06:17 | NaT | 2019-02-01 00:09:44 | 2019-02-01 00:39:56 | 87 | 198 | 6.84 | ... | 2.16 | 0.0 | NaN | 4.0 | 17.070000 | N | Y | N | N | NaN |
5 rows × 24 columns
In preparation for exploring this dataset, let’s apply a few of feature engineering steps. Specifically:
Create a new
tipped
column that indicates if a ride included a tip or not.Create a new
tip_frac
column for the tip amount relative to the overall cost of the ride.Replace the
hvfhs_license_num
column with a more familiarservice
column with names like"uber"
instead of codes like"HV0003"
.
df["tipped"] = df["tips"] != 0
df["tip_frac"] = df["tips"] / (df["base_passenger_fare"] + df["tolls"] + df["bcf"] + df["sales_tax"] + df["congestion_surcharge"].fillna(0) + df["airport_fee"].fillna(0))
df["service"] = df["hvfhs_license_num"].map({
"HV0003": "uber",
"HV0005": "lyft",
"HV0002": "juno",
"HV0004": "via",
})
df = df.drop(columns="hvfhs_license_num")
df.head()
dispatching_base_num | originating_base_num | request_datetime | on_scene_datetime | pickup_datetime | dropoff_datetime | PULocationID | DOLocationID | trip_miles | trip_time | ... | tips | driver_pay | shared_request_flag | shared_match_flag | access_a_ride_flag | wav_request_flag | wav_match_flag | tipped | tip_frac | service | |
---|---|---|---|---|---|---|---|---|---|---|---|---|---|---|---|---|---|---|---|---|---|
0 | B02867 | B02867 | 2019-02-01 00:01:26 | 2019-02-01 00:02:55 | 2019-02-01 00:05:18 | 2019-02-01 00:14:57 | 245 | 251 | 2.45 | 579 | ... | 0.0 | 7.480000 | Y | N | N | N | NaN | False | 0.000000 | uber |
1 | B02879 | B02879 | 2019-02-01 00:26:08 | 2019-02-01 00:41:29 | 2019-02-01 00:41:29 | 2019-02-01 00:49:39 | 216 | 197 | 1.71 | 490 | ... | 2.0 | 7.930000 | N | N | N | N | NaN | True | 0.227015 | uber |
2 | B02510 | <NA> | 2019-02-01 00:48:58 | NaT | 2019-02-01 00:51:34 | 2019-02-01 01:28:29 | 261 | 234 | 5.01 | 2159 | ... | 0.0 | 35.970001 | N | Y | N | N | NaN | False | 0.000000 | lyft |
3 | B02510 | <NA> | 2019-02-01 00:02:15 | NaT | 2019-02-01 00:03:51 | 2019-02-01 00:07:16 | 87 | 87 | 0.34 | 179 | ... | 3.0 | 5.390000 | N | Y | N | N | NaN | True | 0.374532 | lyft |
4 | B02510 | <NA> | 2019-02-01 00:06:17 | NaT | 2019-02-01 00:09:44 | 2019-02-01 00:39:56 | 87 | 198 | 6.84 | 1799 | ... | 4.0 | 17.070000 | N | Y | N | N | NaN | True | 0.147438 | lyft |
5 rows × 26 columns
We’re now ready to explore this dataset in more detail.
Explore#
Let’s analyze our dataset to answer a few questions about tipping practices and driver pay.
As a first step, let’s load the dataset into our cluster’s distributed memory using df.persist()
.
This loads and caches the dataset on the cluster, allowing us to avoid repeated expensive data loading steps when doing multiple computations on the same dataset.
df = df.persist()
Tipping frequency#
How often do New Yorkers tip?
df["tipped"].mean().compute()
0.15895858700806212
About 16% of the time.
Broken down by carrier#
Do ride-share services get tipped at different rates?
df.groupby("service").tipped.mean().compute()
service
juno 0.084400
lyft 0.191230
uber 0.149856
via 0.092949
Name: tipped, dtype: float64
Lyft riders tip more often at a frequency of 19% than Uber riders at 15%.
Tipping amount#
Do riders of different services tip different amounts?
df = df.set_index("pickup_datetime").persist()
import matplotlib.pyplot as plt
ax = plt.subplot()
df2 = df.loc[df["tipped"]]
df2.loc[df2["service"] == "uber", "tip_frac"].resample("1w").median().compute().plot(ax=ax)
df2.loc[df2["service"] == "lyft", "tip_frac"].resample("1w").median().compute().plot(ax=ax)
plt.legend(["Uber", "Lyft"])
plt.title("How much do riders tip?")
plt.xlabel("Time")
plt.ylabel("Tip [%]");
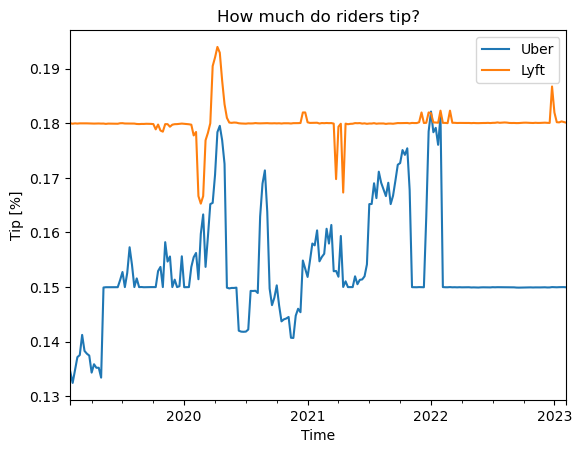
We can see Lyft rider tip relatively consistently at ~18%, while Uber rider’s tip amount is more volatile and generally less than Lyft riders.
Driver Pay#
Let’s also take a look at how much drivers make relative to how much passengers pay.
df.base_passenger_fare.sum().compute()
15818734000.0
df.driver_pay.sum().compute()
12794474000.0
Riders spent about $
16 Billion over the range of the dataset, and Drivers took home almost all of that (about $
13 Billion). Let’s see how that tracks over time.
import matplotlib.pyplot as plt
ax = plt.subplot()
df.base_passenger_fare.resample("1w").sum().compute().plot(ax=ax)
df.driver_pay.resample("1w").sum().compute().plot(ax=ax)
plt.legend(["Base Passener Fare", "Driver Pay"])
plt.xlabel("Time")
plt.ylabel("Weekly Revenue ($)");
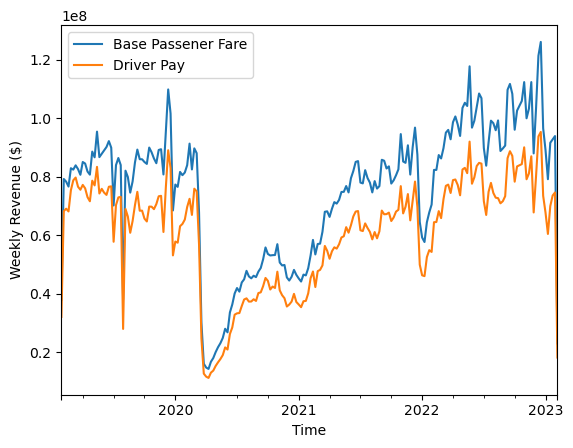
We see some big events, like the massive drop in ridership during COVID-19 and a smaller drop when the Omicron variant was first detected, along with an eventual recovery to greater-than-pre-COVID levels.
We can also see how, ever since the financial outlook of Uber/Lyft style companies has become a bit less friendly, they’ve started to ask for a larger share of revenue from their rides.
(df.driver_pay.resample("1w").sum() / df.base_passenger_fare.resample("1w").sum()).compute().plot()
plt.title("How much of a fare do drivers take home?")
plt.xlabel("Time")
plt.ylabel("Percentage (%)");
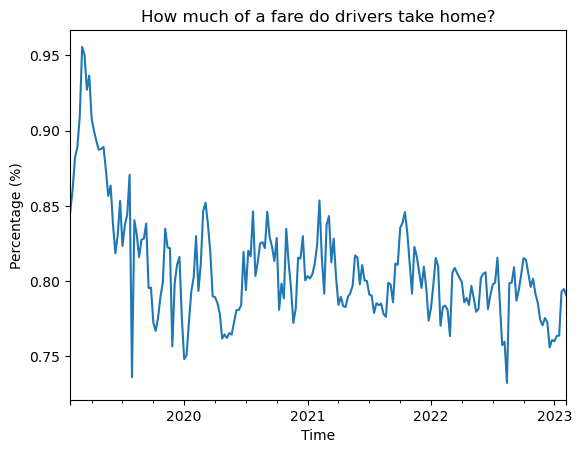
What’s next?#
The Uber/Lyft Rides dataset is complex and rich. What other questions do you want to answer?
Conclusion#
Dask DataFrame makes it easy to analyze a larger-than-memory cloud dataset using the familiar pandas
API. Coiled makes it easy to scale up computing resources by creating large Dask clusters on the cloud with just a few lines of code.
If you have a cloud account it’s easy to try this out yourself. The data is public and this computation costs less than $0.10 in AWS cloud costs. You can sign up under the Coiled free tier here (setup takes a couple minutes).