All Posts
Airflow, Dask, & Coiled: Adding Big Data Processing to Your Cloud Toolkit
- 12 November 2024
Nov 12, 2024
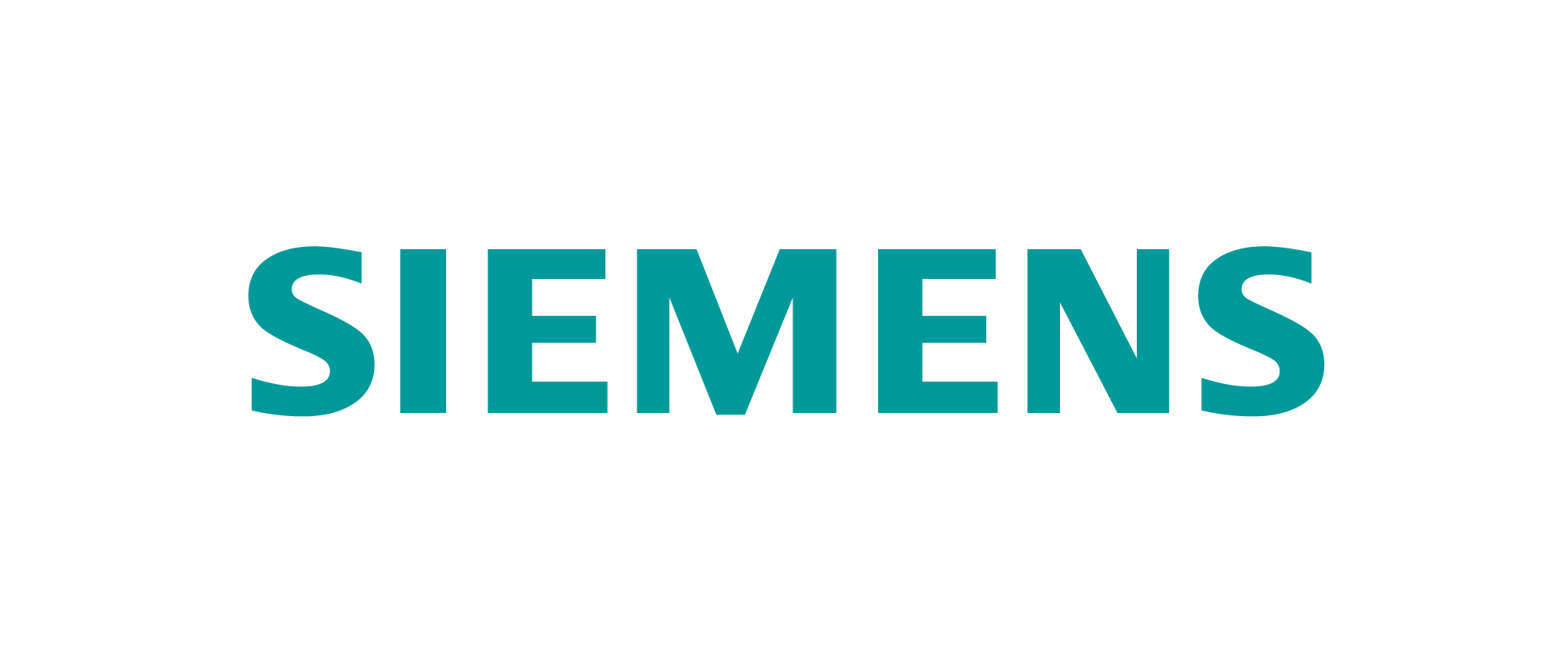
Large Scale Geospatial Benchmarks: First Pass
- 16 October 2024
We implement several large-scale geo benchmarks. Most break. Fun!
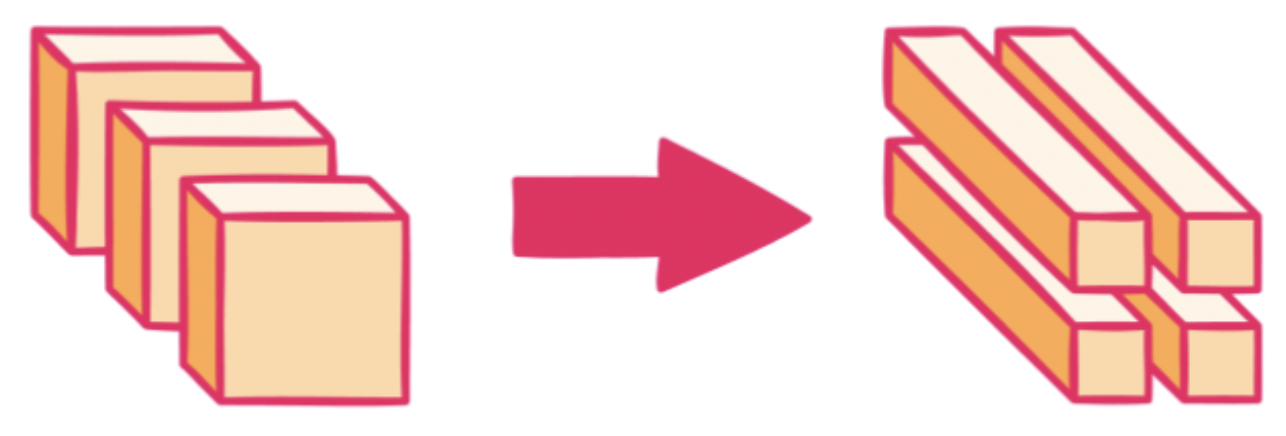
Easy Scalable Production ETL
- 08 April 2024
We show a lightweight scalable data pipeline that runs large Python jobs on a schedule on the cloud.
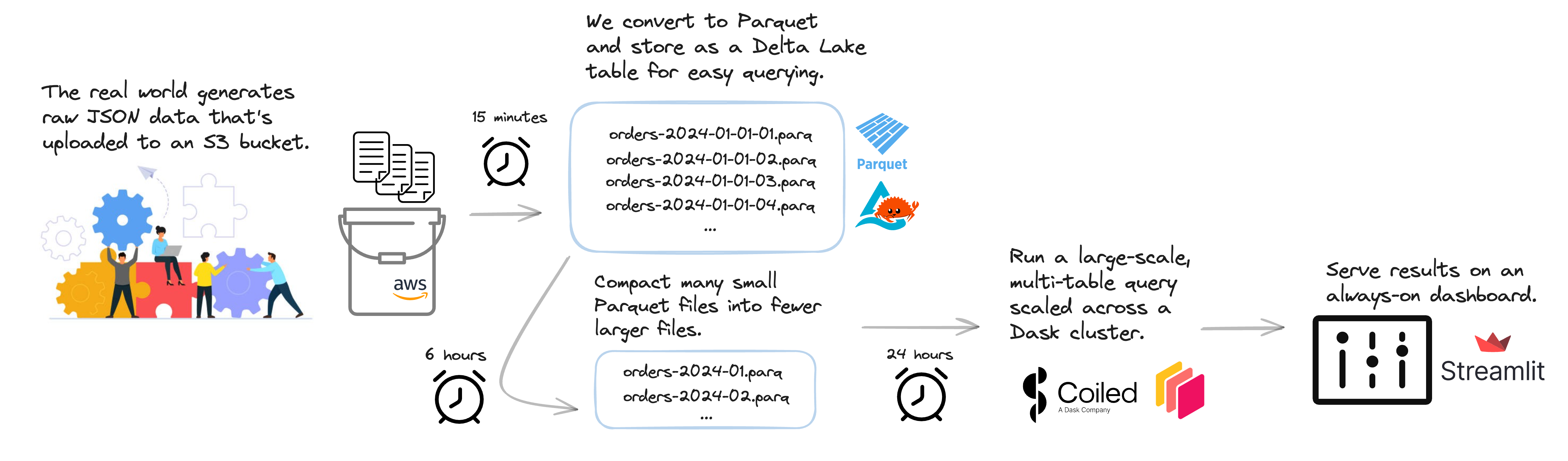
Processing Terabyte-Scale NASA Cloud Datasets with Coiled
- 01 November 2023
We show how to run existing NASA data workflows on the cloud, in parallel, with minimal code changes using Coiled. We also discuss cost optimization.
TPC-H Benchmarks for Query Optimization with Dask Expressions
- 05 October 2023
Dask-expr is an ongoing effort to add a logical query optimization layer to Dask DataFrames. We now have the first benchmark results to share that were run against the current DataFrame implementation.
Coiled observability wins: Chunksize
- 19 September 2023
Distributed computing is hard, distributed debugging is even harder. Dask tries to simplify this process as much as possible. Coiled adds additional observability features for your Dask clusters and processes them to help users understand their workflows better.
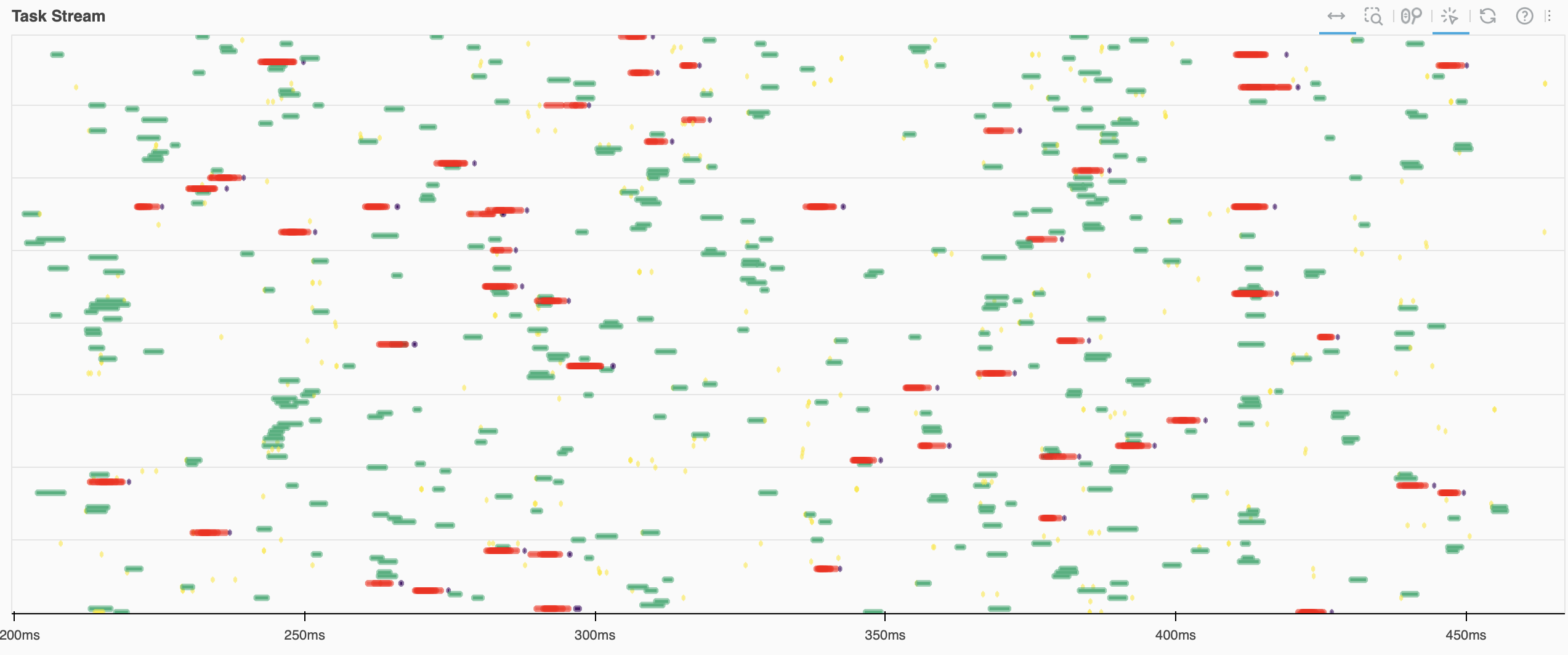
Processing a 250 TB dataset with Coiled, Dask, and Xarray
- 05 September 2023
We processed 250TB of geospatial cloud data in twenty minutes on the cloud with Xarray, Dask, and Coiled. We do this to demonstrate scale and to think about costs.
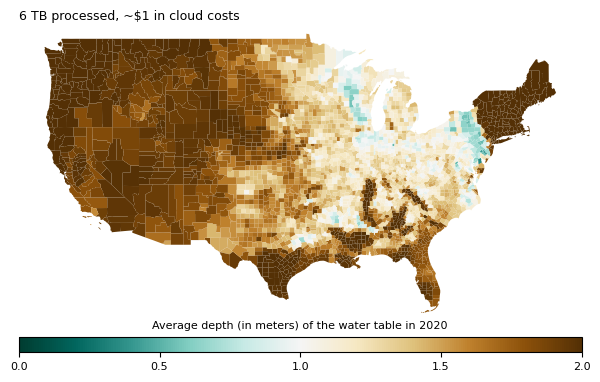
Reduce training time for CPU intensive models with scikit-learn and Coiled Functions
- 01 September 2023
Sep 1, 2023
Fine Performance Metrics and Spans
- 23 August 2023
While it’s trivial to measure the end-to-end runtime of a Dask workload, the next logical step - breaking down this time to understand if it could be faster - has historically been a much more arduous task that required a lot of intuition and legwork, for novice and expert users alike. We wanted to change that.
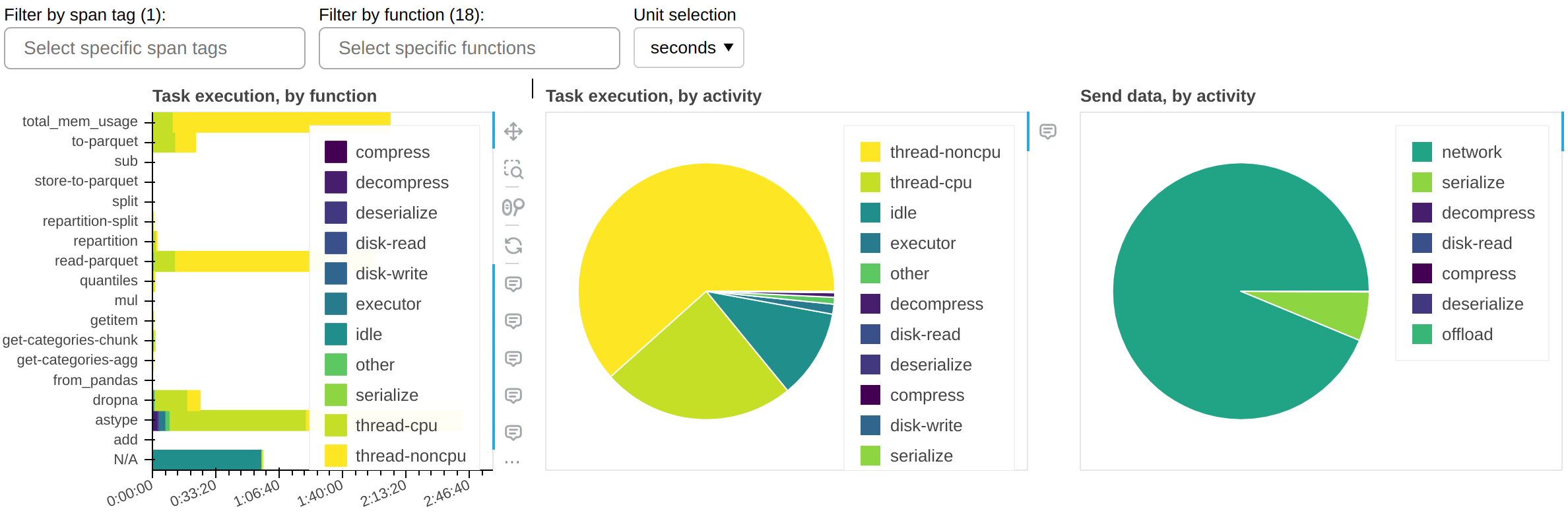
Data-proximate Computing with Coiled Functions
- 10 August 2023
Coiled Functions make it easy to improve performance and reduce costs by moving your computations next to your cloud data.
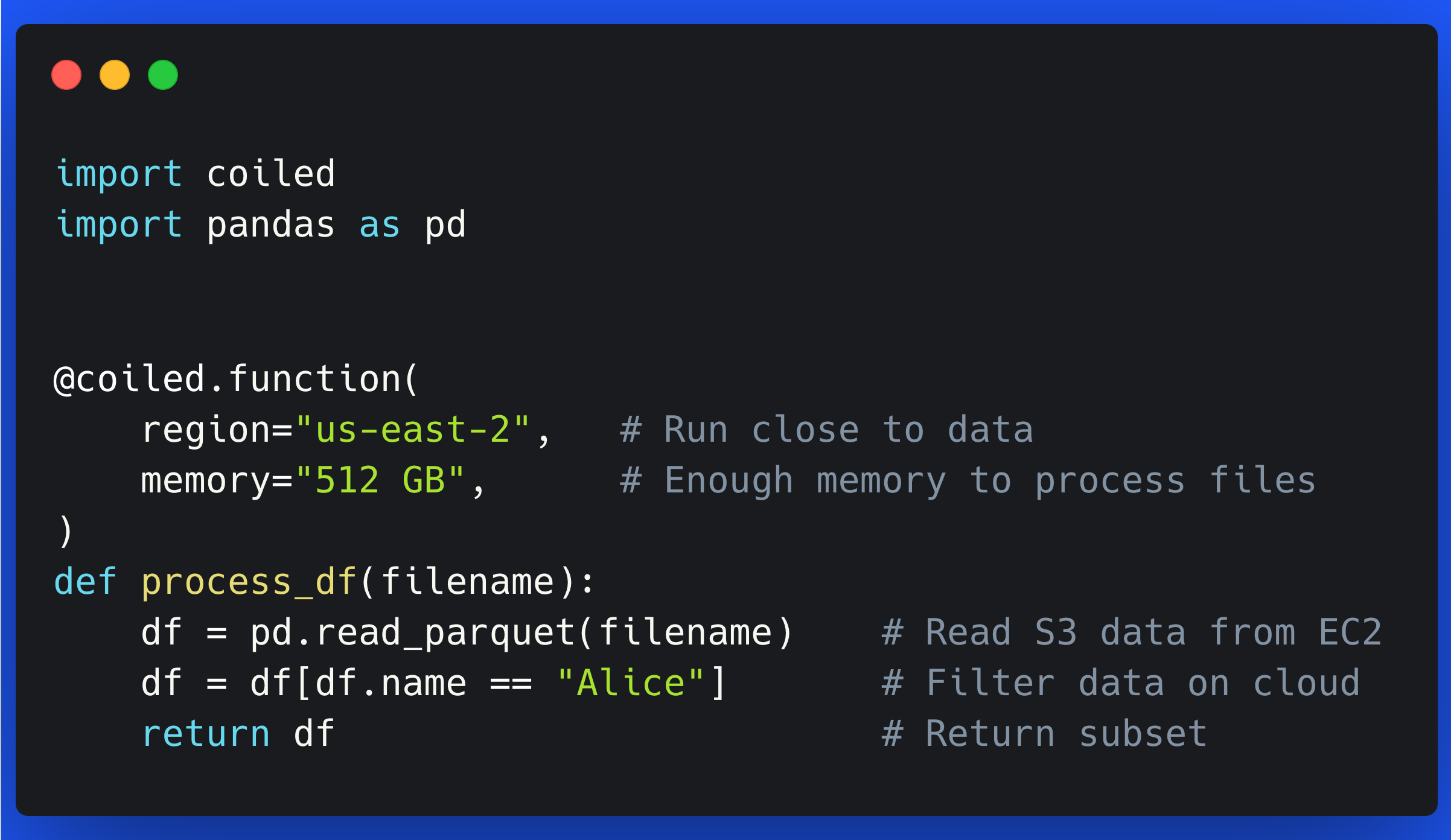
Dask, Dagster, and Coiled for Production Analysis at OnlineApp
- 09 August 2023
We show a simple integration between Dagster and Dask+Coiled. We discuss how this made a common problem, processing a large set of files every month, really easy.
High Level Query Optimization in Dask
- 04 August 2023
Dask DataFrame doesn’t currently optimize your code for you (like Spark or a SQL database would). This means that users waste a lot of computation. Let’s look at a common example which looks ok at first glance, but is actually pretty inefficient.
Easy Heavyweight Serverless Functions
- 01 August 2023
What is the easiest way to run Python code in the cloud, especially for compute jobs?
Coiled notebooks
- 14 June 2023
We recently pushed out a new, experimental notebooks feature for easily launching Jupyter servers in the cloud from your local machine. We’re excited about Coiled notebooks because they:
Distributed printing
- 18 May 2023
Dask makes it easy to print whether you’re running code locally on your laptop, or remotely on a cluster in the cloud.
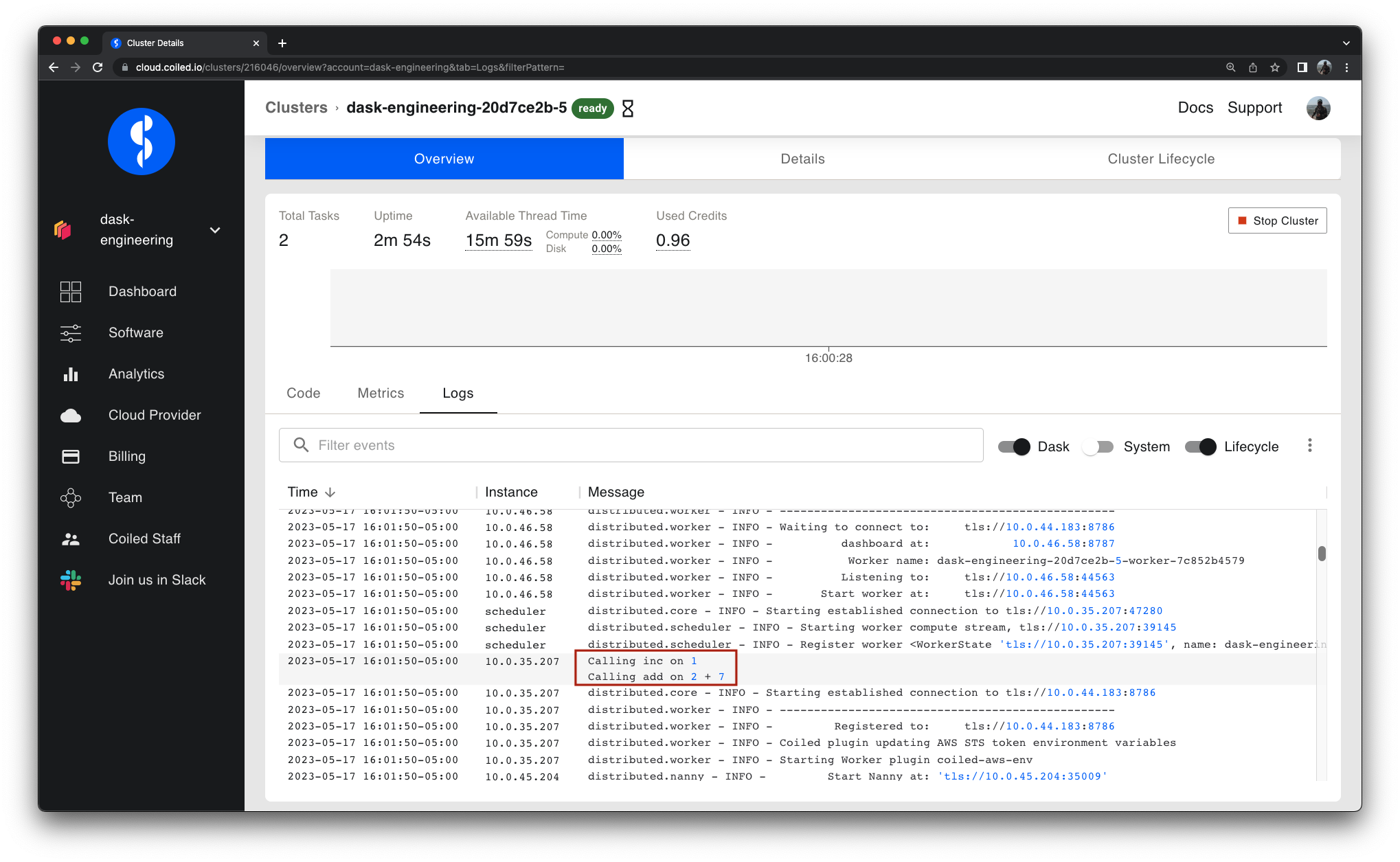
Performance testing at Coiled
- 05 May 2023
At Coiled we develop Dask and automatically deploy it to large clusters of cloud workers (sometimes 1000+ EC2 instances at once!). In order to avoid surprises when we publish a new release, Dask needs to be covered by a comprehensive battery of tests — both for functionality and performance.
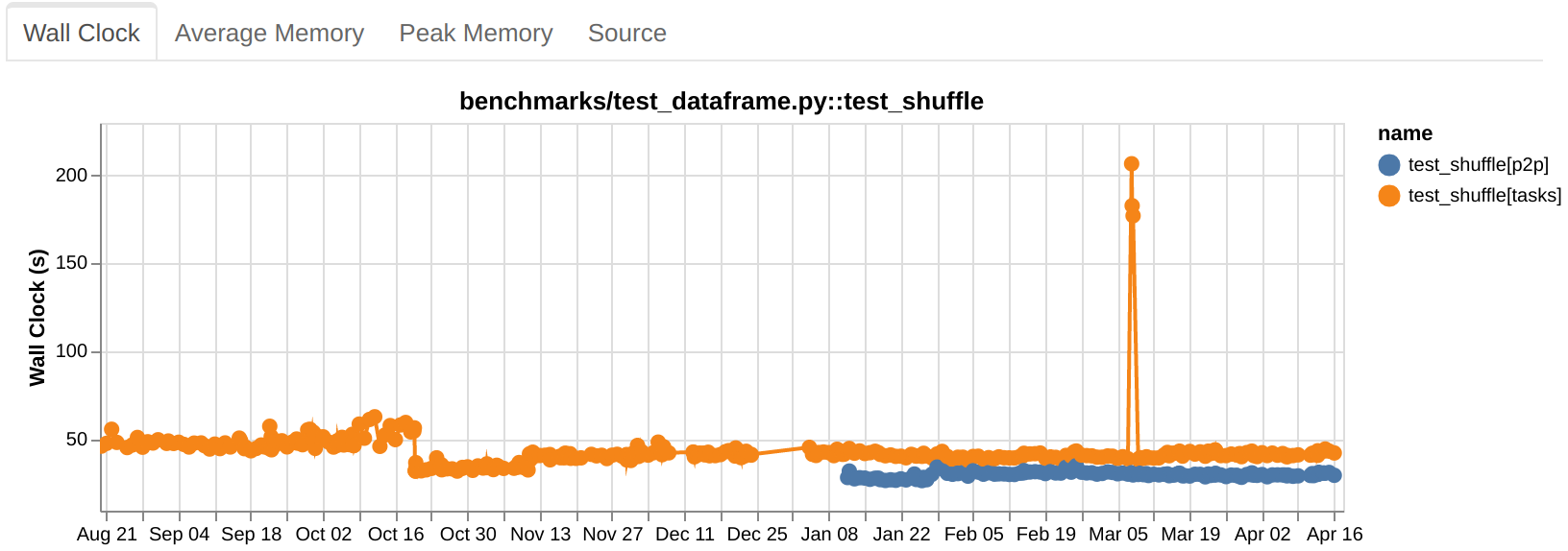
Upstream testing in Dask
- 18 April 2023
Dask has deep integrations with other libraries in the PyData ecosystem like NumPy, pandas, Zarr, PyArrow, and more. Part of providing a good experience for Dask users is making sure that Dask continues to work well with this community of libraries as they push out new releases. This post walks through how Dask maintainers proactively ensure Dask continuously works with its surrounding ecosystem.
Burstable vs non-burstable AWS instance types for data engineering workloads
- 04 April 2023
Apr 4, 2023
How many PEPs does it take to install a package?
- 17 January 2023
A few months ago we released package sync, a feature that takes your Python environment and replicates it in the cloud with zero effort.
Scaling Hyperparameter Optimization With XGBoost, Optuna, and Dask
- 06 January 2023
XGBoost is one of the most well-known libraries among data scientists, having become one of the top choices among Kaggle competitors. It is performant in a wide of array of supervised machine learning problems, implements scalable training through the rabit library, and integrates with many big data processing tools, including Dask.
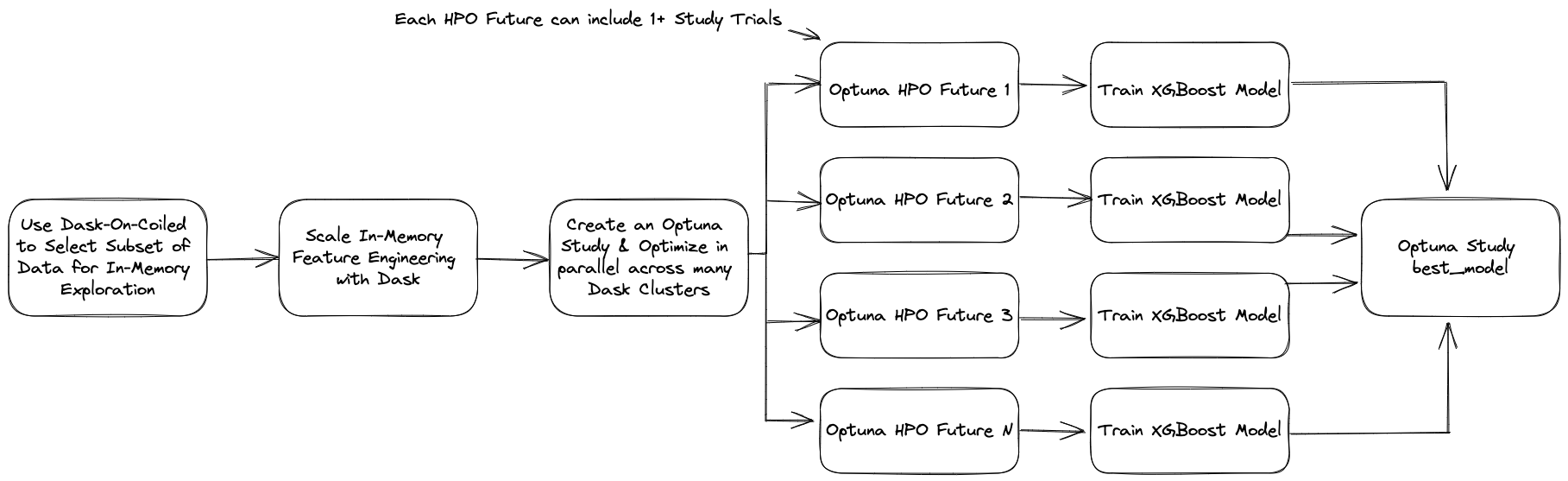
Handling Unexpected AWS IAM Changes
- 06 January 2023
The cloud is tricky! You might think the rules that determine which IAM permissions are required for which actions will continue to apply in the same way. You might think they’d apply the same way to different AWS accounts. Or that if these things aren’t true, at least AWS will let you know. (I did.) You’d be wrong!
Automated Data Pipelines On Dask With Coiled & Prefect
- 19 December 2022
Dask is widely used among data scientists and engineers proficient in Python for interacting with big data, doing statistical analysis, and developing machine learning models. Operationalizing this work has traditionally required lengthy code rewrites, which makes moving from development and production hard. This gap slows business progress and increases risk for data science and data engineering projects in an enterprise setting. The need to remove this bottleneck has prompted the emergence of production deployment solutions that allow code written by data scientists and engineers to be directly deployed to production, unlocking the power of continuous deployment for pure Python data science and engineers.
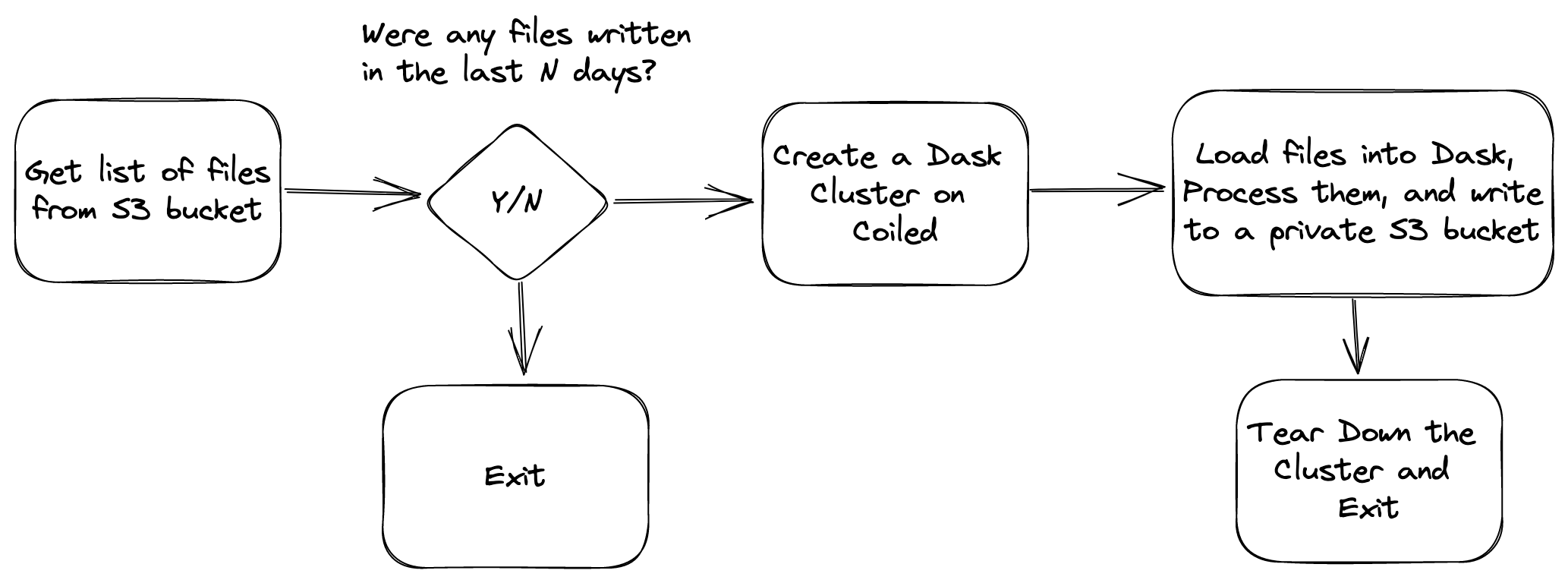
Writing Parquet Files with Dask using to_parquet
- 01 January 2022
This blog post explains how to write Parquet files with Dask using the to_parquet method.
Why we passed on Kubernetes
- 01 January 2022
Kubernetes is great if you need to organize many always-on services and have in-house expertise, but can add an extra burden and abstraction when deploying a single bursty service like Dask, especially in a user environment with quickly changing needs.
Use Mambaforge to Conda Install PyData Stack on your Apple M1 Silicon Machine
- 01 January 2022
Running PyData libraries on an Apple M1 machine requires you to use an ARM64-tailored version of conda-forge. This article provides a step-by-step guide of how to set that up on your machine using mambaforge.
Understanding Managed Dask (Dask as a Service)
- 01 January 2022
Dask is a flexible Python library for parallel and distributed computing. There are a number of ways you can create Dask clusters, each with their own benefits. In this article, we explore how Coiled provides a managed cloud infrastructure solution for Dask users, addressing:
Tackling unmanaged memory with Dask
- 01 January 2022
TL;DR: unmanaged memory is RAM that the Dask scheduler is not directly aware of and which can cause workers to run out of memory and cause computations to hang and crash.
Speed up a pandas query 10x with these 6 Dask DataFrame tricks
- 01 January 2022
Updated April 18th, 2024: For Dask versions >= 2024.3.0, Dask will perform a number of the optimizations discussed in this blog post automatically. See this demo for more details.
Spark to Dask: The Good, Bad, and Ugly of Moving from Spark to Dask
- 01 January 2022
Apache Spark has long been a popular tool for handling petabytes of data in analytics applications. It’s often used for big data and machine learning, and most organizations use it with cloud infrastructure to run models and build algorithms. Spark is no doubt a fast analytical tool that provides high-speed queries for large datasets, but recent client testimonials tell us that Dask is even faster. So, what should you keep in mind when moving from Spark to Dask?
Snowflake and Dask: a Python Connector for Faster Data Transfer
- 01 January 2022
Snowflake is a leading cloud data platform and SQL database. Many companies store their data in a Snowflake database.
Seven Stages of Open Software
- 01 January 2022
This post lays out the different stages of openness in Open Source Software (OSS) and the benefits and costs of each.
Setting a Dask DataFrame index
- 01 January 2022
This post demonstrates how to change a DataFrame index with set_index and explains when you should perform this operation.
Search at Grubhub and User Intent
- 01 January 2022
Alex Egg, Senior Data Scientist at Grubhub, joins Matt Rocklin and Hugo Bowne-Anderson to talk and code about how Dask and distributed compute are used throughout the user intent classification pipeline at Grubhub!
Scikit-learn in the Cloud with Coiled
- 01 January 2022
You can train scikit-learn models in parallel using the scikit-learn joblib interface. This allows scikit-learn to take full advantage of the multiple cores in your machine (or, spoiler alert, on your cluster) and speed up training.
Scale your data science workflows with Python and Dask
- 01 January 2022
Data Scientists are increasingly using Python and the Python ecosystem of tools for their analysis. Combined with the growing popularity of big data, this brings the challenge of scaling data science workflows. Dask is a library built for this exact purpose - making it easy to scale your Python code, and serve as a toolbox for distributed computing!
Repartitioning Dask DataFrames
- 01 January 2022
This article explains how to redistribute data among partitions in a Dask DataFrame with repartitioning…
Reducing memory usage in Dask workloads by 80%
- 01 January 2022
There’s a saying in emergency response: “slow is smooth, smooth is fast”.
Reduce memory usage with Dask dtypes
- 01 January 2022
Columns in Dask DataFrames are typed, which means they can only hold certain values (e.g. integer columns can’t hold string values). This post gives an overview of DataFrame datatypes (dtypes), explains how to set dtypes when reading data, and shows how to change column types.
Perform a Spatial Join in Python
- 01 January 2022
This blog explains how to perform a spatial join in Python. Knowing how to perform a spatial join is an important asset in your data-processing toolkit: it enables you to join two datasets based on spatial predicates. For example, you can join a point-based dataset with a polygon-based dataset based on whether the points fall within the polygon.
Introducing the Dask Active Memory Manager
- 01 January 2022
Historically, out-of-memory errors and excessive memory requirements have frequently been a pain point for Dask users. Two of the main causes of memory-related headaches are data duplication and imbalance between workers.
How to Merge Dask DataFrames
- 01 January 2022
This post demonstrates how to merge Dask DataFrames and discusses important considerations when making large joins.
How to Convert a pandas Dataframe into a Dask Dataframe
- 01 January 2022
In this post, we will cover:
How Coiled sets memory limit for Dask workers
- 01 January 2022
While running workloads to test Dask reliability, we noticed that some workers were freezing or dying when the OS stepped in and started killing processes when the system ran out of memory.
Filtering Dask DataFrames with loc
- 01 January 2022
This post explains how to filter Dask DataFrames based on the DataFrame index and on column values using loc.
Enterprise Dask Support
- 01 January 2022
Along with the Cloud SaaS product, Coiled sells enterprise support for Dask. Mostly people buy this for these three things:
Easily Run Python Functions in Parallel
- 01 January 2022
When you search for how to run a Python function in parallel, one of the first things that comes up is the multiprocessing module. The documentation describes parallelism in terms of processes versus threads and mentions it can side-step the infamous Python GIL (Global Interpreter Lock).
Dask on GCP
- 01 January 2022
Dask is a flexible Python library for parallel and distributed computing. There are a number of ways you can create Dask clusters, each with their own benefits. In this article, we explore how Coiled provides a managed cloud infrastructure solution for deploying Dask on Google Cloud, addressing:
Dask on Azure
- 01 January 2022
Dask is a flexible Python library for parallel and distributed computing. There are a number of ways you can create Dask clusters, each with their own benefits. In this article, we explore how Coiled provides a managed cloud infrastructure solution for deploying Dask on Azure, addressing:
Dask on AWS
- 01 January 2022
Dask is a flexible Python library for parallel and distributed computing. There are a number of ways you can create Dask clusters, each with their own benefits. In this article, we explore how Coiled provides a managed cloud infrastructure solution for deploying Dask on AWS, addressing:
Dask for Parallel Python
- 01 January 2022
Dask is a general purpose library for parallel computing. Dask can be used on its own to parallelize Python code, or with integrations to other popular libraries to scale out common workflows.
Dask and the PyData Stack
- 01 January 2022
The PyData stack for scientific computing in Python is an interoperable collection of tools for data analysis, visualization, statistical inference, machine learning, interactive computing and more that is used across all types of industries and academic research. Dask, the open source package for scalable data science that was developed to meet the needs of modern data professionals, was born from the PyData community and is considered foundational in this computational stack. This post describes a schematic for thinking about the PyData stack, along with detailing the technical, cultural, and community forces that led to Dask becoming the go-to package for scalable analytics in Python, including its interoperability with packages such as NumPy and pandas, among many others.
Dask Read Parquet Files into DataFrames with read_parquet
- 01 January 2022
This blog post explains how to read Parquet files into Dask DataFrames. Parquet is a columnar, binary file format that has multiple advantages when compared to a row-based file format like CSV. Luckily Dask makes it easy to read Parquet files into Dask DataFrames with read_parquet
.
Creating Disk Partitioned Lakes with Dask using partition_on
- 01 January 2022
This post explains how to create disk-partitioned Parquet lakes using partition_on
and how to read disk-partitioned lakes with read_parquet
and filters
. Disk partitioning can significantly improve performance when used correctly.
Cost Savings with Dask and Coiled
- 01 January 2022
Coiled can often save money for an organization running Dask. This article goes through the most common ways in which we see that happen.
Convert Large JSON to Parquet with Dask
- 01 January 2022
You can use Coiled, the cloud-based Dask platform, to easily convert large JSON data into a tabular DataFrame stored as Parquet in a cloud object-store. Start off by iterating with Dask locally first to build and test your pipeline, then transfer the same workflow to Coiled with minimal code changes. We demonstrate a JSON to Parquet conversion for a 75GB dataset that runs without downloading the dataset to your local machine.
Coiled, one year in
- 01 January 2022
Coiled, a Dask company, is about one year old. We’ll have a more official celebration in mid-February (official date of incorporation), but I wanted to take this opportunity to talk a little bit about the journey over the last year, where that has placed us today, and what I think comes next.
Code Formatting Jupyter Notebooks with Black
- 01 January 2022
Black is an amazing Python code formatting tool that automatically corrects your code.
Cloud ETL automation with Github Actions and Coiled
- 01 January 2022
Github Actions let you launch automated jobs from your Github repository. Coiled lets you run your Python code in the cloud. Combining the two gives you lightweight workflow orchestration for heavy ETL (extract-transform-load) jobs that can run in the cloud without any complicated infrastructure provisioning or DevOps.
Better Shuffling in Dask: a Proof-of-Concept
- 01 January 2022
Updated May 16th, 2023: With release 2023.2.1, dask.dataframe introduced this shuffling method called P2P, making sorts, merges, and joins faster and using constant memory. Benchmarks show impressive improvements. See our blog post.
Accelerating Microstructural Analytics with Dask and Coiled
- 01 January 2022
In this article, we will discuss an interesting use case of Dask and Coiled: Accelerating Volumetric X-ray Microstructural Analytics using distributed and high-performance computing. This blog post is inspired by the article published in Kitware Blog and corresponding research.